Sense-Decide-Act: Super Powers
We live in sense-decide-act cycles, technology gives us super powers. .
Welcome back - hope everybody had a great summer! My Africa trip to Kenya and Tanzania was mindblowing. More on that another time.
In this edition, I’m thinking about how as technology improves, it can run the entire gamut of sense-analyse-decide-act cycles without human intervention, but to greatly improve outcomes for human systems.
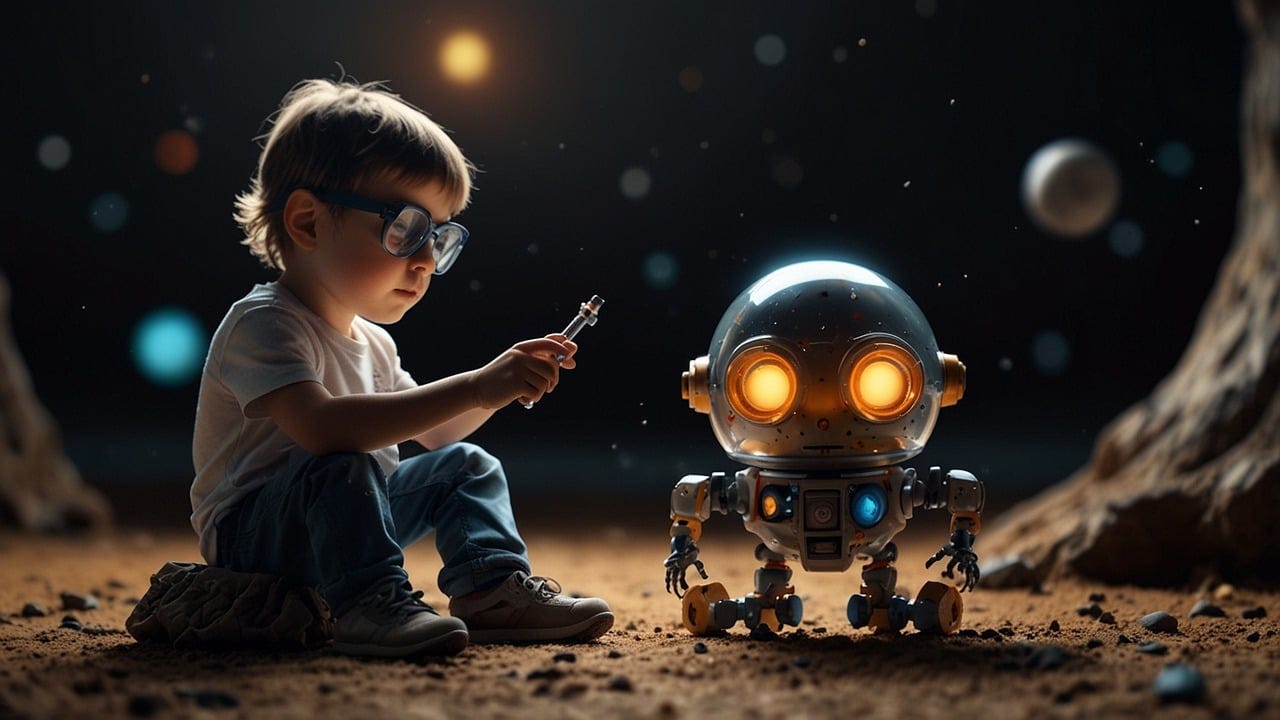
It’s normal for us to think about technologies in isolation - for example we could be speaking about AI, Robotics, Blockchain, Quantum, and many others. While it’s possible to have deep and meaningful discussions about any of these areas, it comes with the hidden challenge that these are not distinct technologies when applied in most contexts, and that from an execution or problem solving perspective we shouldn’t fall into the trap of single-technology tunnel vision.
How do we solve problems? The way our brains are wired, we usually go through cycles of sensing —> decisions —> action. You’re driving along the road, you see a potential hazard ahead - a child playing with a ball, you sense the possible problem, you decide that this could require a sudden stop, you take action - you slow down beforehand. The most critical variable here is often time. Sometimes this cycle happens very quickly, and may even be involuntary such as your instinctive response to touching something hot. The cycle still goes through but it’s might be a different part of your nervous system that kicks in, and your decision making in this case is an example of reflex action - something that actually bypasses your brain and is determined by your spinal cord. The popular decision making cycle OODA (Observation, Orientation, Decision, Action), oft used in business lexicon, was created by the US Airforce for running this cycle extremely quickly in the conscious brain, and under pressure. Daniel Kahneman’s ‘Thinking Fast and Slow’ talks also about the ways in which time and the ‘two brains’ act through this cycle.
Which brings me to the role of technology. While AI has dominated our recent conversations about technology, it’s instructive to think about technology through the sense-decide-act cycle.
Super Powers
Over the past few years, the sophistication of sensing technologies has somewhat gone under the radar, but our ability to sense has increased exponentially. These include solid state LiDAR and Quantum Dot Sensors for visual sensing, wearable and CRISPR based sensors for biosensing, miniaturisation of MEMS and gas sensors, and much more. So the our ability to see, smell, hear, touch or taste things is greatly enhanced thanks to sensing technologies. Because here’s the thing: great as our brains are, our sensory capabilities are actually quite limited, even within the animal kingdom.
All this sensing creates voluminous and varied amounts of data for us to make better decisions, which of course is where AI comes in. Whether you look at autonomous vehicles, or customer chatbots, AI is fundamentally decision automation tool. The benefits of AI in areas ranging from radiology to cybersecurity are well documented. We are very much in the early days of AI and debates about General Intelligence (AGI) and Super Intelligence (ASI) are raging as we speak.
Which brings us to the third leg of our tripod - doing. For our purposes, we can break this up into two streams - digital and physical. Digital actions include things like automated promotions you might see on Amazon, or summarizing a block of text, or generating images. These are currently also being done through software. In fact software has been used for actioning things even before AI. Much of traditional software looks to enable digital actioning but without the use of AI. We simply parameterise the criteria and have humans choose options which the software enables. Like running a batch process for month end accounts in an ERP, or applying a template to a presentation. Today, the coupling of AI with software allows us to do much more sophisticated things at speed and scale. For example generative AI can look at a webpage and generate the code for it. Or a system can identify when a user is struggling to find something on an ecommerce website and offer to help via a chatbot.
But only a small part of our actions are digital. The bulk of what we do is in the real world. And this is where the recent advancements in robotics is very exciting. I wrote in more detail about the robotics revolution recently. But every day I see small and big advancements. Such as the bug robot which has great potential in disaster response scenarios.
The 3 Gaps - Why We Need Help
In the way we’ve traditionally approached this as humans, we might have experienced challenges or gaps at each of the three points.
The first is a sensing gap. We may not be able to see, sense, or hear everything that needs to be noticed. A very good example of this is the challenge of the ‘blind spot’ when you’re driving a car. We lack sensing capabilities in many areas - from early warnings about our health, to adverse weather events. Or whether our ageing family members are well. And in general, we can only sense what our limited range of vision, hearing, and other sensory capabilites allow us. We make up for extremely limited sensory capability compared to the rest of the animal kingdom by better decision making and information processing.
The second gap is the decision gap, or the cognitive gap - we are unable to make process data streams to take decisions with the necessary speed or volumes at which we might need them to be done. In our example of driving, consider the sudden actions of more than one other entity on the road that need increasingly quick decision making. Many accidents happen because our reaction time is a fraction too long, this is partly a decision gap. Also, think about areas such as the complexity of drug discovery and testing, or the challenges of a nurse in A&E ward with multiple patients recovering from complex post operative care. Or even the challenges faced by frontline employees dealing with complex customer complaints.
The last gap is the action gap - even if we take the right decisions, we may not be act on them effectively because of physical challenges. In our automobile example, even if you take the right decision, are you always able to execute in time? That reaction time problem is also partly an action gap. Maybe If all these 3 gaps were close to zero, for a start we might be able to avoid all car accidents. Other action gaps can involve physical limitations - such as shelves being too high for stocking, or objects being too heavy to lift in a warehouse, or spaces being too small or too hazardous to get into.
In a way, the broader purpose of technology is to help us close these 3 gaps. To sense, decide, and act better, faster, and with more effectiveness. The combination of sensors, AI, and robotics, gives us a hugely effective trifecta to achieve this. In fact, with edge technologies, we can replicate the working of reflex actions - where the central brain is not engaged and decisions can be made faster and more locally.
Complex Actions
When we think of sense-decide-act - we need to bear in mind that it’s not one cycle but in fact, a series of rapid cycles. Every action leads to more sensing, and in everyday real world scenarios, the environment and conditions keep changing in ways that as humans we are used to but for tech based systems involves learning. Even to navigate the aisle of a department store, a robot designed for shelf stocking must continuously scan for people in the aisles, as well as empty spots on shelves. And every decision to move in a particular direction involves further scanning for people moving in that area and repeatedly going through the decision-action cycles. (Assuming this is being done when humans are also shopping or working in the area).
Historically therefore humans have had to step in to complete this loop as technology has only been able to do parts of this but without being able to complete a full learning loop or adjust the approach in real time. We are now getting to a stage where tech can actually run this loop independently of human support, creating self reinforcing cycles of learning and exploration. Here are 2 examples.
Digital Twins
In the world of complex and evolving decisions, as the series of decisions we make impact how the future pans out, the challenge of getting it right gets tricker. This is the realm of digital twins, as they allow us to test a load of what if scenarios and effectively ‘rehearse the future’ as my futurist colleagues like to call it. For example Formula E drivers can’t practice in their cars because ePrix races are run in city centres - so they use simulators to rehearse the track and the races before the race. Pilots train in simulators too.
These are expensive devices designed for very specific experiences, but increasingly the vast combinations of sensors and AI are allowing us to create digital twins of human organs, business processes, or critical infrastructure or assets which can also be put through a series of what-if scenarios so that their futures can also be rehearsed. Rolls Royce already does this with aircraft engines, and we’re learning to do this with cities and complex systems. My colleagues at TCS have already built digital twins of human hearts and human skin. The picture below is an abstraction of how divergent scenarios can emerge from the same starting point, based on our actions or those of others, requiring very different responses from us as the situation evolves. You could think of the green picture as a happy-path - where things evolve as we want them to, and the purple one as a scenario where things are spinning out of control so your actions have to be increasingly defensive/ corrective.
Humanoid Robots
Another area where this comes together is with the current generation of humanoid robots. Robots, as you know are subject to the Moravec’s paradox, which says that AI and Robots find many things easy that we find incredibly hard, but the converse is also true, simple acts like negotiating a staircase to find a glass and fill it with water from a tap can be a very complex task for a robot. But with every year, this is getting better, thanks to all the advanced robotics you’ve probably seen from companies like Boston dynamics. I wrote an earlier post about the advancements in robotics, but the combination of robotics and AI can give robots a next generation brain, and decision making ability that they might have lacked so far. Robots that can sense, decide, and act in ways that respond to the world around them would make for a very interesting humanoid species - we might even be approaching uncanny valley!
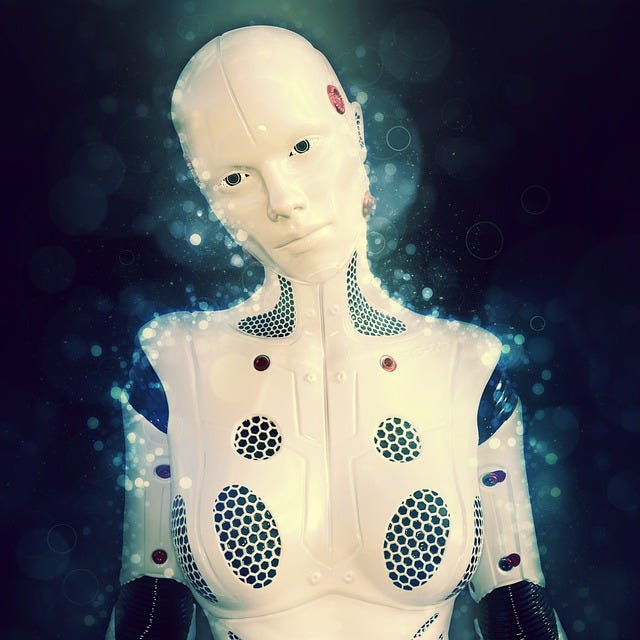
AI Reading
AGI - What exactly is AGI - Melanie Mitchel’s work explores what exactly constitutes AGI. My personal take is that intelligence as problem-solving is one axis, however broad the range of problems are. Building a value system that is infused with ethicality, morality, and all the other inputs that allow humans to choose between outcomes is a different axis altogether, and I’ve not seen any work yet, that tells me AI systems are being trained in this manner. (Science.org, via Azeem Azhar)
AI Agents perform 40% tasks - but when they do, they do so using 3% of the cost of a human baseline. (Exponential View - Azeem Azhar)
Trends: McKinsey Tech Trends: amongst other things, this report shows applied AI and Generative AI as two distinct areas of evolution, along with a host of others.
What is AI: Here’s one more comprehensive read about what AI is, does, and can potentially do. Including reflections from many luminaries such as Turing on what exactly is ‘thinking’. The piece includes a great example of how rewards can skew AI outputs - such as the use of the San Francisco bridge in a recipe for spaghetti and meatballs! (MIT Tech Review)
Other Reading
Energy Transition: Solar & Storage costs are now lower than cost of any other energy sources in Germany
Systems Thinking: Here’s an interest example of how enlarging the problem helped in the case of the recent Russian Prisoner Exchange.
Money: How Digital Money Really Works: Here’s a good blog to follow about how digital money really works.
Olympics - Budget Busters: a simple picture tells you a story about olympics hosting costs in the last 30 years vis a vis their budgets. It would appear that the more expensive the plan, the more likely it is to go over the budget!
Thanks and enjoy the sunshine, see you soon!